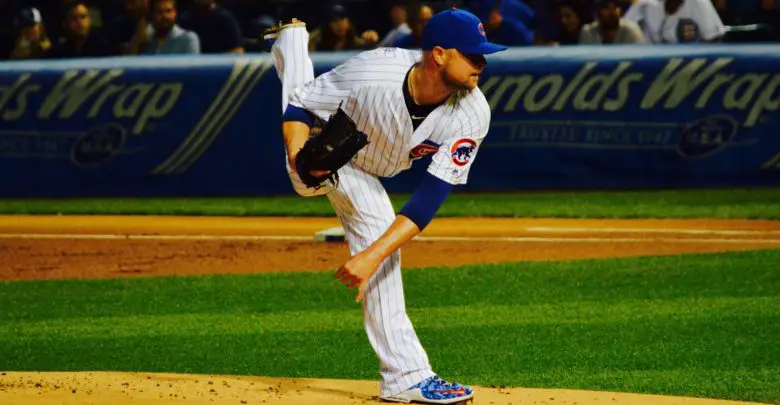
Predicting Spin Rate of Jon Lester’s Cutter May Improve Analysis, Even Performance
The Same Holds True for Other Cubs Pitche(r)s
Previously the domain of Maytag and Whirlpool, spin rate has been established as part of baseball’s analytical vernacular over the last few years. Just as it’s statistical brethren like exit velocity and launch angle have changed the way hitters are viewed, spin rate — expressed in terms of RPM — is a way to measure a pitch’s efficacy.
That’s obviously a very imperfect definition and it’s not a simple matter of the highest spin rate generating the best results, but there are some basic truths we can establish. Just as a higher exit velo will generally result in more hits, a higher spin rate will generally yield pitches that are harder to hit.
What I’d like to do here is offer a brief tutorial on spin rate and why it’s important in our analysis, after which I’ll get into some specifics in terms of Jon Lester’s best pitch. And I’m talking about some really exciting stuff, folks, even if you’re not necessarily into the newfangled metrics.
Why spin rate is important
Simply put, spin rate is a pitcher’s currency. Spinning the ball at a higher rate correlates to more whiffs and weaker contact, so a pitcher with a high spin rate is probably getting pretty good results and is going to make money. There are instances, however, when a pitcher generates a high spin rate with one or more pitches, but he hasn’t been successful overall for some reason.
Take Charlie Morton, for example. Astros executives apparently signed Morton because of his exceptional curve spin rate, thinking they could bring out the most in his arm. The former Pirates righty went on to become an integral part of a World Series championship at a fraction of what he was truly worth. So if spin rate is a pitcher’s currency, the ability to identify those pitchers who are ready to best leverage their talent is where baseball executives make their money.
But what if we could identify the factors that lead to exceptional spin rate on a pitcher-by-pitcher basis? Front offices could use this knowledge to better predict which pitchers are primed for a Morton-like turnaround based on quality spin rates that may not have been consistent enough for overall success. Then these pitchers could work with their coaches to understand and improve those contributing factors, and to correct them when they go awry.
With that in mind, I created a model to predict spin rate using individual pitcher traits. Each model will vary from pitcher to pitcher, but the foundational aspects of each will not deviate. We can predict spin rate by using release point (exactly where he lets go of the ball) and extension (the distance between release point and the pitching rubber, or how much closer the ball is to the plate). Each trait exclusively correlates to spin rate, so combining them in one model can explain almost a third of what makes a pitcher spin the ball. Adding in velocity explains more of the variance, but I wanted to focus solely on mechanics.
And while 30 percent may not seem like a lot, explaining over one-third of anything in this goofy, random sport is pretty significant.
Predicting spin rate of Jon Lester’s cutter
Jon Lester is only as successful as his cutter, which he throws roughly once out of every four pitches. Anything that messes with that pitch is problematic, so if coaches can identify the elements that make Lester’s cutter move more, they could target specific changes during side sessions.
Lester’s extension and horizontal release point explain about 31 percent of what makes his cutter move. There is less than a 0.000000000000000022 percent chance that release extension doesn’t actually influence spin, and it’s less than 0.0000000000000364 percent for horizontal release point. When combined, the chances that both release extension and horizontal release point don’t do anything to spin drop to less than [insert a lot of zeroes before some random numbers] percent.
Based on this low chance of flukiness, we have a great degree of confidence that coaches could use release point and extension data to “fix” Lester’s cutter when spin rate decreases.
Now, I understand that what I just wrote may mean nothing to most folks reading it, so let’s illustrate the effect of release point and extension on the spin rate of Lester’s cutter to provide additional context. Each dot in the graph below is one cutter from the 2017 season. As you follow the blue line from left to right, notice how spin rate RPMs increase. Also, notice how the line dips low as spin goes up.
This shows us the combined effect extension and release point have on spin rate. Specifically, when Lester is more extended, his spin rate is lower. And when the veteran southpaw lets go of the cutters closer to his body, that signature pitch is likely to spin more.
There’s also a weird cluster of pitches on the top right of the graph. This is probably because Lester’s release point was extremely close to his body in those instances, further increasing his spin rate.
The key for Lester is finding a release point closer to his body and with limited extension. Let’s say his cutter is getting shellacked at some point next season because his spin rate is lower and the pitch isn’t moving as much as it needs to. Making an adjustment to decrease his extension and get his release point closer to his glove side could yield better results, even in real-time.
Final thoughts
Because spin rate is one of the newer fads in today’s stats world, we’re really only beginning to understand exactly how to understand and apply it. But we saw what happened with Morton, and Theo Epstein even mentioned that the Cubs signed Tyler Chatwood in part because of his exceptional spin rate. It’s only logical, then, that we should look for things that impact a pitch’s RPMs, as we’ve done for Lester above.
Like any baseball metric, this isn’t perfect. While I’m pretty happy that we can explain nearly one-third of what makes Lester’s cutter spin, there is still a big chunk of unquantifiable influence in there. As such, I advise you to interpret with caution. With more practice and better data, however, the validity of our results will continue to increase.
And this isn’t just about Lester. We can build similar models to the one above for other Cubs pitchers, as well. That way, once the 2018 season starts, we can track mechanics and results in real-time and see if any mechanical changes may be leading to more or less spin. In other words, we can see why a pitcher might be struggling or performing well.
See, I told you this was exciting.
Stat Notes
I ran a multivariate linear regression (spin rate ~ release extension + release_pos_x). I then plotted the residuals vs. the coefficients. Both extension and horizontal release point predicted spin rate (p < 3.64E-13 and p <2.2E-16, respectively; combined p < 2.2E-16; adjusted R squared = 0.308). Missing values were excluded from the model. Data was retrieved using the baseballR package in weekly increments from the 2017 season. Code available upon request.