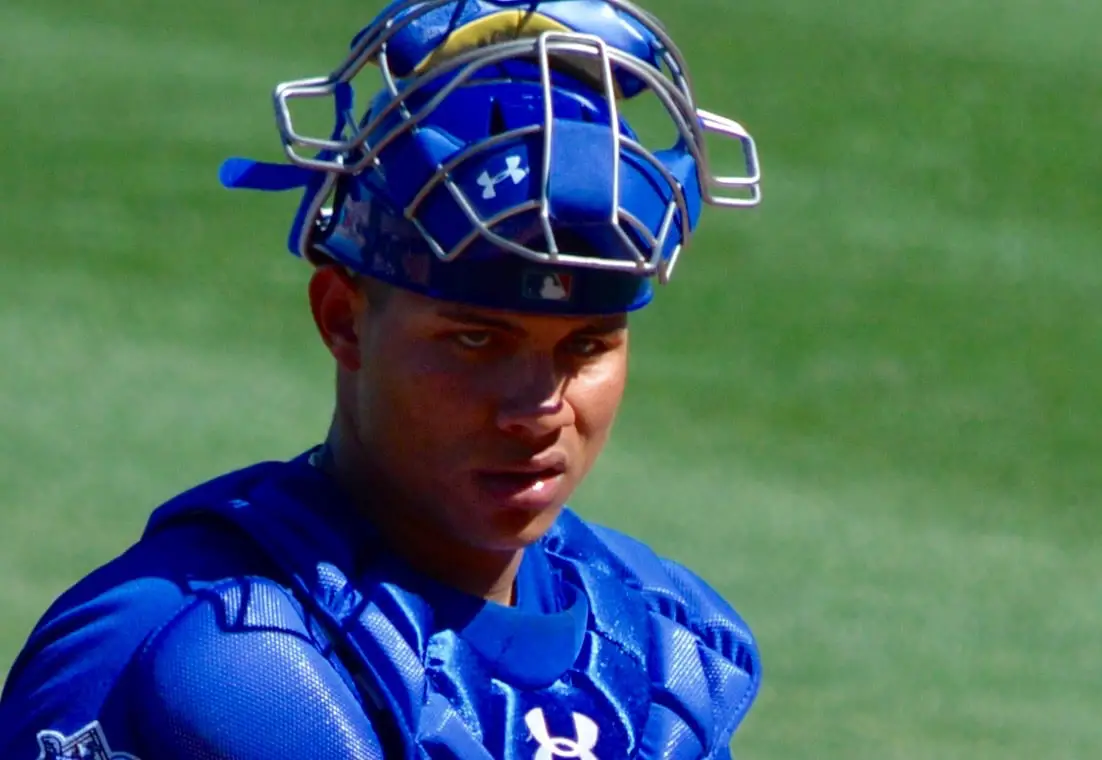
Did 2016 Projections Accurately Predict the Cubs?
We’re inundated with various projection models during the offseason, each with a unique spin on forecasting players. It figures that some are going to be better than others. ZiPS and Steamer, for example, are featured on FanGraphs and updated as the season rolls along. But how good are these projections models? Did they predict last season with a good degree of accuracy?
I went back and charted qualified Cubs players’ 2016 ZiPS and Steamer weighted on base average (wOBA) projections against their actual 2016 stats.
ZiPS/Steamer vs. Reality (wOBA) | ||||
Player | ZiPS | Steamer | ZiPS/Steamer Average | 2016 Stats |
Willson Contreras | 0.303 | 0.297 | 0.300 | 0.363 |
Tommy La Stella | 0.313 | 0.315 | 0.314 | 0.333 |
Miguel Montero | 0.298 | 0.310 | 0.304 | 0.299 |
Matt Szczur | 0.296 | 0.280 | 0.288 | 0.305 |
Kris Bryant | 0.370 | 0.370 | 0.370 | 0.396 |
Jorge Soler | 0.335 | 0.326 | 0.331 | 0.333 |
Javier Baez | 0.330 | 0.323 | 0.326 | 0.316 |
Jason Heyward | 0.349 | 0.347 | 0.348 | 0.282 |
Dexter Fowler | 0.340 | 0.325 | 0.332 | 0.367 |
David Ross | 0.232 | 0.253 | 0.243 | 0.326 |
Ben Zobrist | 0.346 | 0.336 | 0.341 | 0.360 |
Anthony Rizzo | 0.375 | 0.380 | 0.377 | 0.391 |
Addison Russell | 0.316 | 0.303 | 0.310 | 0.316 |
Overall, Steamer and ZiPS did an okay job of forecasting players’ seasons. The correlation between 2016 projections and reality was a mild .49 (.1-.3 = weak, .4-.6= mild, .7-.99= strong), meaning that the systems were able to capture approximately 24% of what actually happened.
Projections wildly underestimated Willson Contreras, Dexter Fowler, Kris Bryant, and David Ross, while overestimating Jason Heyward. Forecasted relatively accurately, however, were Javy Baez, Addison Russell, Jorge Soler, Miguel Montero, Tommy La Stella, Matt Szczur, and Anthony Rizzo.
The fine writers at Beyond the Box Score graded the major prediction models’ accuracy in 2016 (see below) and determined Steamer was the best at forecasting wOBA. ZiPS actually performed terribly in 2016, finishing near the bottom in every major offensive category.
How do we interpret these projections, then? The takeaway here is that ZiPS and Steamer miss about 75% of what goes into a baseball season, largely because the computers simply can’t account for major mechanical changes. For example, Willson Contreras and Kris Bryant’s dramatic swing changes resulted in overshooting their prognoses. Unless baseball statisticians develop a deep learning model, perhaps named Theo, we will never know whether a projection will hold true. Yet, despite the shortcomings of ZiPS and Steamer, both do a modest job at predicting numbers in the face of baseball’s natural randomness.